Diversity in the study of data: four Fondecyt will enhance the work of IMFD researchers
January, 2024. The National Agency for Research and Development (ANID) announced the results of the Fondecyt Regular 2024, the program that is positioned as the most central public policy in the science and innovation system for the development of basic science in the country.for the development of basic science in the country. This year, there are five projects carried out by researchers from the Millennium Institute Foundational Research on Data These projects emphasize the diverse areas of study of the IMFD, addressing studies related to data in its most diverse aspects: from privacy, the behavior of query languages, analysis of urban data and social networks, the funds of this cycle cover the great diversity of studies carried out at the IMFD.
Queries in graph databases
Domagoj Vrgoč, an academic at the Institute of Mathematical and Computational Engineering of the Catholic University and a researcher at the Millennium Institute Foundational Research on Datawas awarded a four-year grant for the research "New Challenges in Graph Query Answering", a pioneering research project that will study the fundamental properties of the new ISO standard for graph query languages called GQL.
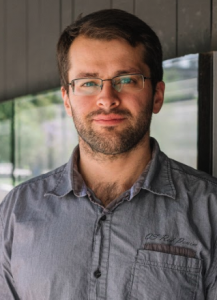
Graph databases offer an intuitive conceptualization: with nodes representing entities and arcs representing the connections between entities. The flexibility and adaptability they provide as a system for representing information has made them one of the fastest growing sectors in the last decade. Their commercial adoption and proliferation of different engines generated a need to create a common language for graph databases.
This has resulted in the Graph Query Language (GQL) standard as well as SQL/PGQ, which extends SQL with capabilities to analyze graph databases. These two standards are the object of study of the IMFD researcher's project, which aims to understand the query language properties shared by both standards, GQL and SQL/PGQ, to understand which fragments of both standards can be efficiently evaluated and to develop efficient evaluation algorithms for both cases.
Health data privacy
A research group that produces many linguistic resources and computational models for spoken Spanish in Chile is the one led by Jocelyn Dunstan Escudero, the academic Mathematical and Computational Engineering Institute of the Universidad Católica and the Department of Computer Science of the Catholic University and researcher at the Millennium Institute Foundational Research on Data.
At Privacy-preserving methods for clinical natural language processing in Spanish, the researcher will seek to study, create and to study, create and evaluate privacy-preserving methods to foster to promote the ethical use of clinical text data in large linguistic modeling (LLM) applications, formally ensuring the protection of sensitive patient data.formally guaranteeing the protection of sensitive patient data. As co-investigators it counts with the academic of the Department of Computer Science of the U. Chile and also IMFD researcher Matías Toro and with the professor of linguistics of the UC Fredy Núñez.
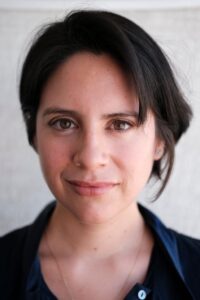
Large linguistic models (LLMs) are revolutionizing the way we interact with machines. For example, a growing community of researchers is interested in new ways in which LLMs, such as ChatGPT, could solve increasingly complex tasks involving unstructured text.
These models require huge amounts of text to train and use hundreds or billions of parameters. Although pre-trained linguistic models work well with less data, the large number of parameters could lead to unwanted memorization of RUTs, names or addresses. unwanted memorization of RUTs, names or addressesmaking them susceptible to privacy attackssuch as inferring whether someone belongs to a dataset..
Medical applications are a promising field for the use of pre-trained LLMs, since it deals with large amounts of free text from electronic medical records, such as diagnoses, prescriptions or inpatient notes.such as diagnoses, prescriptions or inpatient notes. However, privacy preservation inHowever, privacy preservation in medicine is a cornerstone, as exposing sensitive patient information violates human rights.. This objective is crucial, since unstructured text can improve predictive tasks and favor the use of epidemiological information, which can be used in the creation of public policies and guidelines in the health area.
Effects of advertising in social networks
The detection of propaganda in social networks and the characterization of its effects on social networks is the interdisciplinary project of Marcelo Mendoza, academic of the Department of Computer Science of the Catholic University and IMFD researcher who was also awarded the Regular Fondecyt. In this, he will work with a team based on international collaboration and with the support of specialists in the area of communications as the co-investigator of this project Marcelo Santos, from the School of Journalism of the Diego Portales University and Millennium Nucleus for the Study of Politics, Public Opinion and Media in Chile, MEPOP.
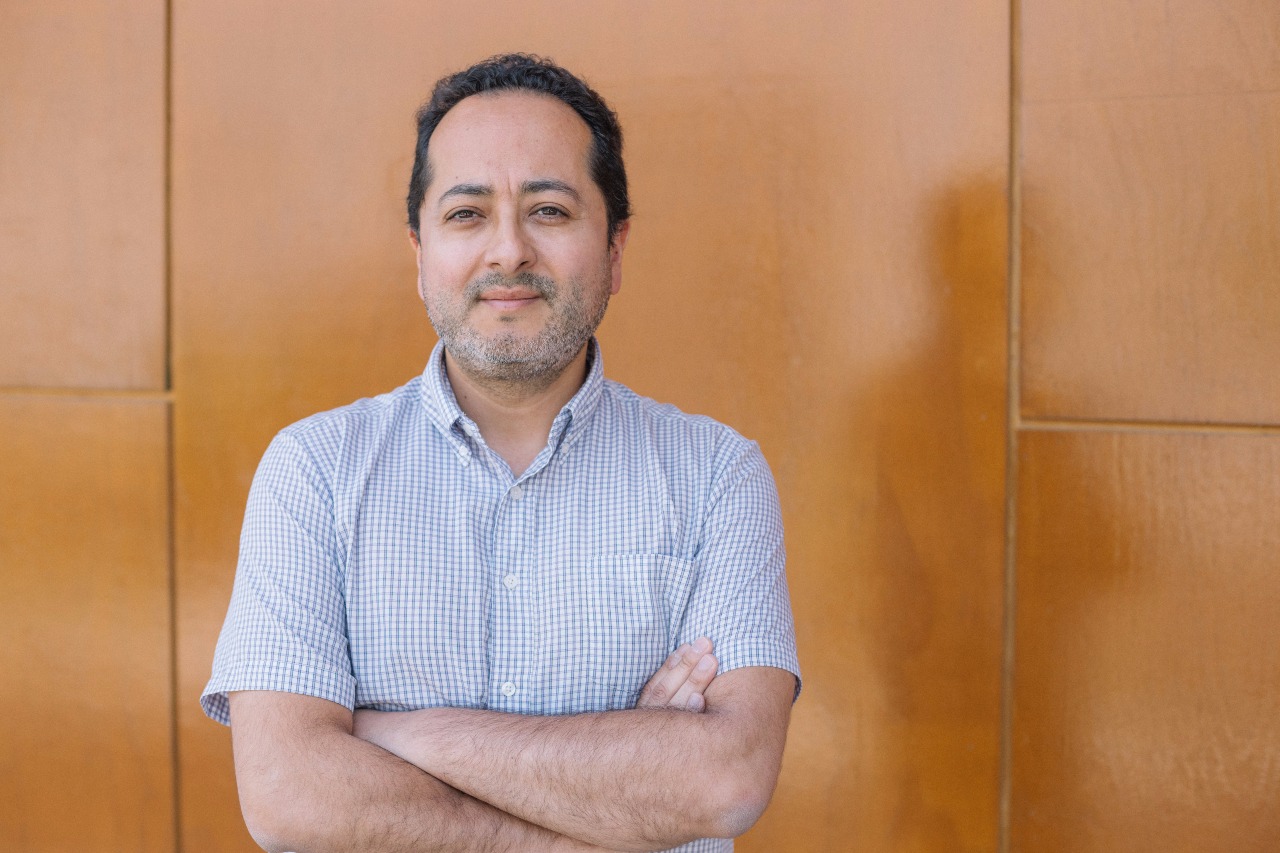
The research team seeks to to analyze whether users reproduce the biases of the press media based on linguistic propaganda strategies, that is, the existence of linguistic contagion in social networks through the use of propaganda strategies. For this purpose, they will analyze the presence of persuasive text in social networksThe detection of the use of propaganda in the writing of news helps to understand how in the information field we can be influenced by reproducing stereotypes. In this work, we will develop techniques will be developed to detect the use of manipulation strategies in news sources, such as the use of stereotypical language and the use of stereotypes.such as the use of stereotyped language, exaggeration or the appeal to fear. The required natural language processing techniques should handle various propaganda strategies and be able to detect their use in real time.
The project seeks to overcome the state-of-the-art results based on an improvement of the data used to train this type of models, which have severe class imbalance. The first models developed in the project consider tackling the problem in English, and then develop models in Spanish, using linguistic model-assisted data augmentation (LLM) strategies, as well as human annotation for infrequent classes. The second stage of the project considers the 2025 presidential election as a case study.
Multimodal data in cities
In "Embed the City: An Artificial Intelligence-based Approach to learn Transferable Representations from Multi-source and Multi-modal Urban Data," Hans Löbel, a scholar in the Department of Computer Science of the Catholic University and the Department of Transportation and Logistics Engineering UC and IMFD researcher, seeks to improve the characterization of urban phenomena, capturing both general characteristics and particular details. The research seeks to overcome the limitations of neural network-based models through the development of a learning framework with explainable models to learn transferable representations from multimodal and multisource urban data.

The main challenge faced today when trying to use the great diversity of data that exists in urban planning is the management of the enormous amounts of multimodal data that cities generate: images, text, sensor data, social network content, surveys, socio-demographic data, geographic information systems (GIS) data: making efficient use of this enormous diversity can be a daunting task and very difficult to tackle.
Neural networks have emerged as powerful tools that are able to address these problems: convolutional neural networks can process large amounts of images, while large language models are able to handle survey texts and social networks.
The research defines three main limitations in the use of these systems for planning and defining public policies: the first is the lack of explainability, which hinders the ability to identify the main factors driving the phenomena, thus undermining their reliability. The second is related to the fact that current models generally focus on individual data analysis, losing the benefits of a joint learning approach that considers multiple perspectives on urban phenomena. And as a third limitation, it is indicated that the classical model training scheme of training and testing is not the most suitable for multimodal data and data from diverse sources, as it tends to overfit the model and hinders the generalization of the task.
To date, these models can only capture general aspects of complex urban environments, and lack the introspective capability needed to derive detailed insights that can support sound urban planning and policy formulation. Here, the proposal of the study is to overcome these limitations by developing a learning framework with explainable models, which allow learning transferable representations from multimodal and multisource urban data, in order to improve the characterization of urban phenomena, capturing both universal characteristics and particular details from different perspectives.
For this, the research was planned in three stages: building explainable neural network models to evaluate factors in image-based urban tasks; learning multimodal representations to comprehensively characterize diverse data sources that capture urban information; and finally developing a pre-training framework to easily transfer urban representations to various downstream tasks.