Noticias
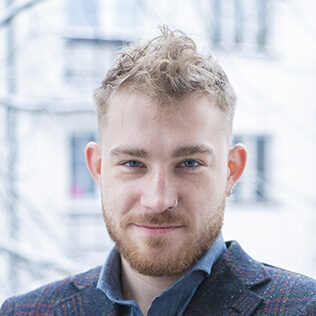

Agosto, 2023.- Desde 1974, expertos en data science, machine learning y procesamiento de lenguaje natural, entre otras áreas, se reúnen en la Conferencia Europea de Inteligencia Artificial (ECAI). La versión 2023 de la cita -considerada como uno de los tres mayores cónclaves del mundo en su área- se realizará en Cracovia (Polonia) y contempla la presentación de diversos papers, entre los cuales estará uno elaborado por investigadores del Instituto de Ingeniería Matemática y Computacional (IMC) de la P. Universidad Católica de Chile e investigadores del Instituto Milenio Fundamentos de los Datos.
El trabajo aceptado para ECAI 2023 -a efectuarse entre el 30 de septiembre y el 5 de octubre- se titula “No Agreement Without Loss: Learning and Social Choice in Peer Review”. Sus autores son Pablo Barceló -director del IMC UC e investigador del Instituto Milenio Fundamentos de los Datos (IMFD)-, Mauricio Duarte -doctor en matemáticas de la U. Andrés Bello-, Cristóbal Rojass -académico IMC UC e investigador del Centro Nacional de Inteligencia Artificial (Cenia)– y Tomasz Steifer, posdoctorando internacional del IMC UC y el IMFD.
Tal como plantean los investigadores en la presentación del paper, en los sistemas de revisión por pares por los que pasan los estudios científicos antes de ser publicados, a menudo “se pide a los revisores que evalúen varias características de los trabajos, como la calidad técnica o su novedad. Una puntuación se asigna a cada una de las características predefinidas y sobre la base de estas, el revisor tiene que proporcionar una evaluación cuantitativa general”. Tomasz Steifer, doctor en ciencia de la computación del Instituto de Ciencia de Computación de la Academia Polaca de Ciencias, explica que en este proceso influyen diferentes factores, que en algunos casos se conocen como “sesgos” y que introducen un elemento de arbitrariedad.
Por ejemplo, indica, el llamado sesgo de conmensuración surge cuando a los revisores “se les pide que evalúen características diferentes e incomparables, como la novedad y la corrección técnica, y que luego unan esas puntuaciones incomparables en una puntuación general. Esta puntuación final suele ser, al mismo tiempo, una recomendación para aceptar o rechazar”. En este sentido, la arbitrariedad proviene del hecho de que los revisores “difieren en cuanto a la importancia que tienen las diferentes características para ellos. Si envío un trabajo que obtiene excelentes puntajes en la función A y una evaluación mediocre en la función B, es posible que tenga suerte y obtenga un revisor que piense que la función A es más importante que la función B. Pero podría tener mala suerte y obtener un puntaje general malo, porque mis revisores prefieren la función B que la función A. Aun así, nos gustaría creer que el proceso de revisión no se trata de tener suerte, sino que sólo de la calidad de un artículo. Es por eso por lo que marcos como el de Noothigattu, Shah y Procaccia ofrecen una promesa muy tentadora de mejorar el sistema de revisión, para que sea menos arbitrario, más justo para los autores y mejor para seleccionar buenos artículos”.
El método de dichos autores, pertenecientes a las universidades de Carnegie Mellon y Harvard, ya fue aplicado el año pasado en la versión número 36 de la conferencia AAAI sobre inteligencia artificial. El fin de los organizadores fue, precisamente, tratar de identificar revisiones que pudieran presentar un sesgo de conmensuración significativo.
Según comenta Steifer, la estrategia propuesta por el equipo de investigadores consiste en “observar cómo los diferentes revisores mapean las puntuaciones de las funciones en una puntuación general y tratan de llegar a una asignación agregada, una especie de asignación ‘promedio’. La pregunta entonces es cómo hacer esta agregación, es decir, qué parámetros usar en su método”. Normalmente, precisa el doctorando del IMC, cuando se propone un método en el área de machine learning o inteligencia artificial éste se prueba empíricamente: “La revisión de pares es distinta porque no tenemos un estándar de información de la más alta calidad con el cual comparar. En realidad, no sabemos el ‘valor real’ de un paper, y la mejor información que tenemos es la que nos entregan los revisores. Así que lo único que estos autores pudieron hacer es presentar un argumento teórico/matemático de por qué su método es bueno”.
En el método de Noothigattu, Shah y Procaccia los autores plantearon tres axiomas. “Estos pueden ser considerados como las propiedades mínimas que un método de agregación debiera tener, y ellos mostraron que entre una variedad de parámetros distintos sólo uno permite satisfacer todos los axiomas al mismo tiempo”, señala el postdoctorando del IMC e IMFD.
Steifer precisa que los investigadores de Carnegie Mellon y Harvard, presentaron algunos argumentos teóricos que explican por qué su método es bueno si los parámetros son bien elegidos. “Por supuesto, al hacer esto, realizaron algunas suposiciones que resultaron ser demasiado simplificadas cuando eran contrastadas con la realidad”, indica. En el paper aceptado en ECAI 2023, el postdoctorando del IMC y sus coautores se enfocaron en “revisar esta teoría y analizar si realmente tiene sentido, una vez que cambiamos sus suposiciones por otras que están más cercanas a lo que ocurre en la vida real. Lo que vimos, entonces, es que su método realmente no tiene todas las buenas propiedades teóricas que ellos afirmaban tener”.
En el trabajo, Steifer y sus coautores comentan que los “resultados obtenidos no revisten sólo un interés teórico, ya que el método en cuestión fue puesto en práctica por los organizadores de una importante conferencia sobre inteligencia artificial”. Por eso, mirando hacia el futuro el postdoctorando del IMC UC y el IMFD señala que varios grupos en importantes universidades están trabajando actualmente en métodos concebidos para lidiar con las problemáticas específicas de la revisión de pares.
“Pienso que recién estamos empezando a entender cómo y hasta qué punto los métodos matemáticos y computacionales pueden ayudarnos a mejorar la revisión de pares. Soy optimista y pienso que, con el tiempo y a medida que crezca nuestra comprensión, seremos capaces de hacer que este proceso sea mejor, más justo y garantice que la buena investigación sea publicada y se propague”, afirma Tomasz Steifer.
Fuente: Comunicaciones IMC UC






