Events
24/Aug
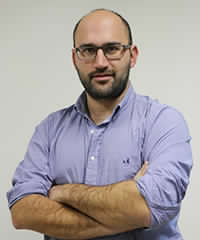

Abstract: The Gaussian process (GP) is a probabilistic model for functions. Unique advantages of the GP are its generality, the fact training and prediction can be performed analytically, and its ability to represent uncertainty. In this talk, we first present the concept of a generative model to introduce the probabilistic perspective to Machine Learning, then, via an intuitive extension of basic generative models we introduce the GP model. We will also show how to train (or adjust) the GP model in the light of observed data, and how it can be used in real-world applications of denoising, prediction, reconstruction and deconvolution of general time series. The talk concludes proposing a novel generative model to address an inherent drawback of GP models: its inability to model non-Gaussian data.
Bio: Felipe Tobar holds an MSc in Electrical Engineering from Universidad de Chile in 2010, and a PhD in Signal Processing from Imperial College London in 2014. He was then with the Machine Learning Group at the University of Cambridge as an Associate Researcher for one year. From August 2015 Felipe has been with Universidad de Chile, as a Research Fellow at the Center for Mathematical Modeling. Felipe‘s research interests lie in the interface between Machine Learning and Statistical Signal Processing and include approximate inference, Gaussian processes, kernel methods, and spectral estimation.
Date: 24 de agosto de 2018, 12.00 a 13.00 horas
Venue: Sala Felipe Flajolet, Departamento de Ciencias de la Computación, U. de Chile