Noticias
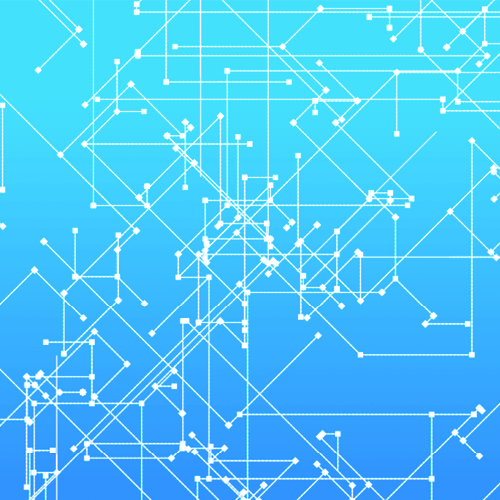

July 2024.- An algorithm to estimate the centrality of nodes in a network, which is competitive in accuracy with machine learning methods, is the innovative proposal that researchers Francisco Plana, PhD in Computer Science from the University of Chile; Andrés Abeliuk, DCC academic and IMFD research collaborator, and Jorge Pérez, former IMFD researcher and co-founder of “cero.ai”, present in the scientific article “Quickcent: a fast and frugal heuristic for harmonic centrality centrality estimation on scale-free networks“. This work was recently published in Computing, a scientific journal published by Springer, focused on computer science and numerical computation.
About this research, Andrés Abeliuk explains: “Centrality indices identify key nodes in a network, such as people or web pages, that are influential or prominent. Centrality evaluates how centrally a node is located based on its proximity to other nodes. For example, a node with high centrality can communicate effectively with many other nodes in the network, facilitating rapid information propagation and strengthening network cohesion.”
Calculating these indexes in networks is computationally expensive and they do not scale efficiently, which limits their application in large networks. The DCC U. Chile academic comments that the work developed together with Francisco Plana and Jorge Perez, “proposes a heuristic based on local indicators, such as the popularity of a node, to estimate the centrality of the nodes in the network. This heuristic achieves high accuracy in networks that show an uneven distribution where a few nodes are highly connected, taking advantage of the correlation between local centrality and global centrality in a network”.
In addition, an algorithm for estimating the centrality of nodes in a network is proposed that is competitive in accuracy with machine learning methods, with the advantage of being efficient in terms of time and computational cost. “This approach suggests that simple heuristics can be effective for estimating measures in complex networks. Particularly in social networks, where the more connected a node is, the more likely it is to receive new links,” concludes Andres Abeliuk.
Source: DCC U. Chile






